Unveiling the Distinguishing Features of AI-Generated Images and Deepfake Images
- Sachin
- May 6, 2024
- 4 min read
AI (Artificial Intelligence) and deepfakes are both fascinating and impactful technologies, but they serve very different purposes.
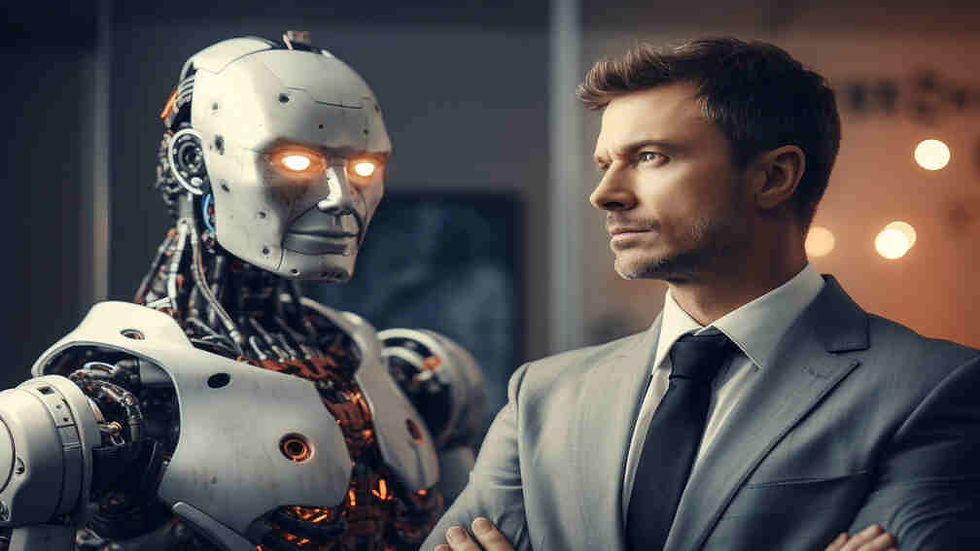
AI (Artificial Intelligence) and deepfakes are both fascinating and impactful technologies, but they serve very different purposes. Let’s delve into each of them:
About AI-Generated Image
AI-generated images are fascinating creations produced by artificial intelligence models. These models learn from vast amounts of data and generate new visual content based on patterns they've observed.
Here's a high-level overview of how AI-generated images work:
1. Generative Models:
AI-generated images are typically created using generative models. These models learn to generate new data (such as images) by analyzing existing examples.
Some popular generative models include:
Variational Autoencoders (VAEs): These models learn to encode input data into a compact representation (the "latent space") and then decode it back into the original data. VAEs can generate new data by sampling from the latent space.
Generative Adversarial Networks (GANs): GANs consist of a generator and a discriminator. The generator creates new data, while the discriminator tries to distinguish between real and generated data. The two components improve each other iteratively.
Autoregressive Models: These models generate data one element at a time, conditioning on previously generated elements. PixelRNN and PixelCNN are examples of autoregressive models.
2. Training Process:
Generative models are trained on large datasets (e.g., millions of images). During training, they learn to capture statistical patterns, textures, and structures.
The loss function guides the model to generate realistic images. For GANs, the generator aims to fool the discriminator, while the discriminator aims to correctly classify real vs. generated images.
Training can take days or weeks, depending on the complexity of the model and available computational resources.
3. Sampling and Creativity:
Once trained, generative models can sample from their learned distribution to create new images.
The creativity of AI-generated images comes from the model's ability to combine and remix features from the training data. It can produce novel combinations that humans might not have imagined.
4. Applications:
AI-generated art: Many artists and designers use generative models to create unique artworks.
Style transfer: Models can blend the style of one image with the content of another.
Image completion: Fill in missing parts of an image.
Super-resolution: Enhance image resolution.
DeepDream: Create surreal and abstract images.
Remember that while AI-generated images can be impressive, they lack intentionality and consciousness. They're the result of statistical patterns, not artistic intent.
About Deepfake Image
A deepfake is a media file—image, video, or speech—that has been deceptively altered using deep neural networks (DNNs) to change a person's identity. These alterations often involve a "face swap," where the likeness of one person is convincingly transferred onto another.
Here are some key points about deepfakes:
1. Definition:
Deepfakes are synthetic media created by digitally manipulating existing content. They can replace one person's likeness with another, making it appear as if the second person is saying or doing something they never actually did.
2. Technology:
Deepfakes use deep learning techniques, which involve training neural networks on large datasets of images or videos. These networks learn to generate new content based on the patterns they observe in the training data.
3. Applications:
Videos: Deepfakes are commonly associated with manipulated videos. For example, a deepfake video could make it seem like Barack Obama called Donald Trump a humorous insult or Mark Zuckerberg confessed to controlling stolen data.
Photos: Deepfake technology can also create entirely fictional photos from scratch. For instance, a non-existent journalist named "Maisy Kinsley" was likely a deepfake, and another LinkedIn profile, "Katie Jones," turned out to be a suspected deepfake created for a foreign spying operation.
Audio: Deepfakes extend beyond visuals. They can create convincing voice clones of public figures, leading to potential voice-based scams.
4. Concerns:
Misuse: While deepfakes have creative and entertainment potential, they raise serious concerns. The majority of deepfakes found online are pornographic, often mapping faces of female celebrities onto porn stars. Additionally, there's a risk of revenge porn fueled by easy-to-create deepfakes.
Weaponization: Deepfake technology can be weaponized against individuals, especially women. It's essential to address the ethical and legal implications of its use.
5. Detection Challenges:
Detecting deepfakes is challenging because they are becoming more convincing. Researchers and technology experts are continually working on improving detection methods, but the arms race between creators and detectors continues.
In summary, deepfakes are a powerful yet controversial technology that blurs the line between reality and fiction. As they become more accessible, it's crucial to stay informed about their implications and potential risks.
Conclusion
AI has a wide range of applications, while deepfakes specifically focus on creating realistic but deceptive media. As technology evolves, responsible use and regulation are crucial to mitigate potential harm and maximize benefits.
Also read:
Comentários